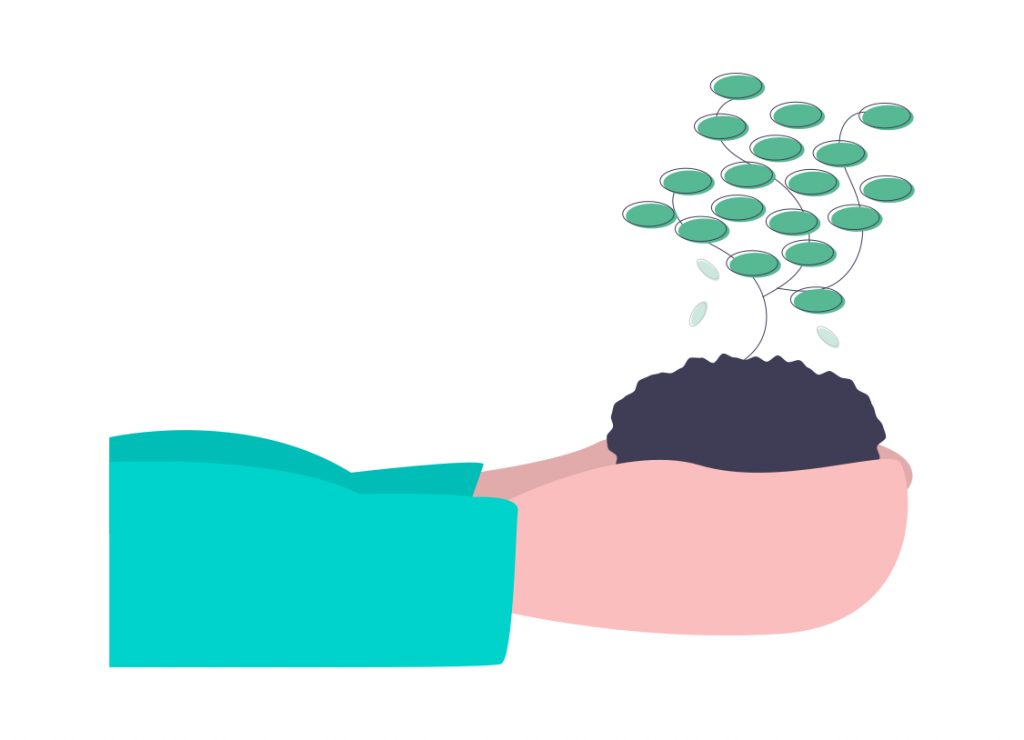
Localizing low-resource languages (LRLs) offers several benefits for both local communities and international businesses. It not only empowers consumers to access information and services in their native language, enhancing their participation in society, but also enables businesses to reach new markets.
However, these languages pose specific challenges when it comes to localization, as they often lack the resources, tools, and infrastructure that are readily available for widely spoken languages. It’s important to recognize the value of linguistic diversity and work toward preserving and promoting them.
In this article, we go over the definition of low-resource languages, sharing examples, as well as discuss the complexity of adapting content to the cultural and linguistic nuances of these languages.
What are low resource languages?
First off, you need to know that machines are trained to learn languages through a process called natural language processing (NLP), which is a subfield of artificial intelligence (AI). The training process involves using large amounts of linguistic data to teach machines the patterns, structures, and semantics of a language.
Low resource languages refer to languages for which there is limited linguistic data and resources available for NLP tasks. In the context of NLP and machine learning, having “low resources” typically means a lack of annotated text, speech data, or other linguistic resources needed to train and develop effective language models.
Therefore, some of the reasons why a language might be considered low resource include having digital presence, lacking annotated datasets, being underrepresented in academic research, or having little computational resources.
Examples of LRLs
While it’s hard to provide an exhaustive list of low-resource languages, some of the languages that are often considered low resource due to factors mentioned above, include:
- Afrikaans
- Albanian
- Amharic
- Basque
- Bengali
- Chichewa
- Galician
- Guarani
- Hindi
- Høgnorsk
- Inuktitut
- Irish
- Kinyarwanda
- Kurdish
- Malay
- Malagasy
- Migmaq
- Minderico
- Nishnaabe
- Quechua
- Sami
- Scottish Gaelic
- Somali
- Swahili
- Tigrinya
- Uralic
- Zulu
As such, these languages may include:
- Many indigenous and minority languages.
- Some regional or less widely spoken languages.
- Languages with limited online content and presence.
Of the approximately 7,000 languages that exist today, nearly a quarter of them are facing the threat of extinction. Given this, it is estimated that nearly 90% of all languages will vanish within the next century. The vast majority of these languages are considered LRLs. However, by utilizing NLP techniques, we can safeguard these languages and their unique writing systems for posterity.
Why are some widely spoken languages considered low resource?
While you may assume that languages that are spoken by a large communities worldwide are high resource languages, this is not exactly true. The designation of a language as low-resource or high-resource is not solely based on the number of speakers, but rather on a combination of factors, as mentioned earlier.
Swahili, for example, is spoken by approximately 200 million people, mainly in East Africa. While efforts are being made to increase the digital presence of Swahili, it is still considered a LRL in terms of online content and available datasets for NLP tasks. The availability of annotated data for Swahili may be limited compared to more widely spoken languages.
Icelandic, on the other hand, is spoken by around 360,000 people, primarily in Iceland. While Icelandic is spoken by fewer people, it may have more annotated data available for certain tasks due to active efforts in linguistic research and development. It also has relatively high digital presence, with a considerable amount of content available online, including literature, news, and educational materials.
Challenges of low-resource languages
The most significant challenge for low-resource languages is the scarcity of high-quality, labeled data. Unlike high-resource languages like English, French, or Spanish, which have vast amounts of text and speech data, LRLs often lack the resources to create and collect sufficient data for language processing models.
This scarcity of data leads to several issues such as reduced performance (e.g. lower accuracy in machine translation), overfitting (when language models may become overly reliant on specific patterns and phrases), errors, and even biases.
The development of language processing technologies for LRLs is often hampered by resource constraints, including lack of expertise, limited funding, and limited infrastructure. The lack of active participation from the communities that speak LRLs can also pose challenges in developing and deploying language processing technologies.
Furthermore, language processing models for LRLs should be developed with sensitivity to the cultural context and nuances of the language. Without the active involvement of community members, it can be challenging to ensure that these models are culturally appropriate and respectful of the language’s heritage.
Addressing the challenges of LRLs
Overcoming the challenges of low resource languages requires the use of techniques like back-translation, synthetic data generation, and data sharing. These can help artificially increase the amount of available data for training LRL models.
Building new dictionaries, corpora, and grammar resources for LRLs can help bridge the data gap and provide the necessary foundations for developing language processing tools. Leveraging knowledge from high-resource languages, such as bilingual data and pre-trained language models, can also improve the performance of LRL systems.
Governments and funding agencies play a significant role in supporting LRL research and development by providing funding, infrastructure, and training programs for language professionals. In addition, collaborating with LRL communities is also crucial for collecting data and ensuring that language processing models are culturally sensitive and appropriate.
Translate high and low resource languages with POEditor
POEditor is a versatile translation management system that empowers users to handle both low and high resource languages effectively. The platform’s capabilities extend beyond the mainstream languages, allowing businesses and individuals to translate content into languages with varying levels of available resources.
The platform offers collaborative features to facilitate efficient translations. Teams of translators can work seamlessly on projects, ensuring accuracy and consistency. This collaborative model is particularly beneficial for low resource languages where a dedicated team of professional translators might be scarce.
To enhance efficiency, POEditor integrates machine translation features, which can be especially useful for low resource languages. While machine translation can provide a starting point, the collaborative nature of the system ensures that the output is refined and validated by human contributors for accuracy and cultural nuances.
To sum it up
Each language and community is unique, so a flexible and adaptive approach is crucial when dealing with low-resource languages in the context of localization. Overcoming the challenges can be a complex task, but through strategies like crowdsourcing, collaborating with local communities, building terminology databases, and using machine translation with human review, it’s possible to make strides in overcoming these challenges.