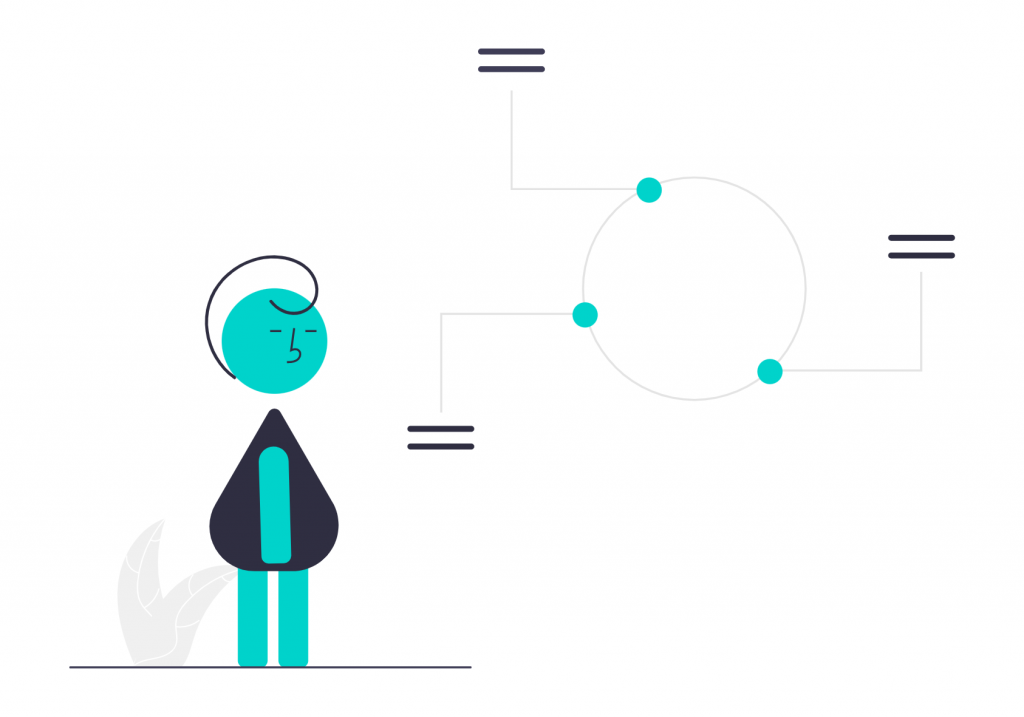
Segmentation is one of the first steps in localization and translation. As the name suggests, it involves breaking down the source text into manageable units, or “segments,” which are the smallest translatable parts that retain their meaning and context. Segmentation in localization is an essential step for many reasons, but we’ll talk about all this—and more—below.
Why segmentation is important
Segmentation allows translators to focus on each piece individually, which benefits both them and the business. A few advantages you can expect include:
- Maintained context
- Improved efficiency
- Enhanced consistency
- Facilitated automation
By dividing content into logical parts, translators can focus on each piece individually. This reduces the risk of errors and improves the overall quality of the work. A well-segmented content ensures that each part of the text can be translated without losing its contextual relevance.
Translators and editors often work with large volumes of text, and breaking this content into smaller units makes the process more manageable. And it doesn’t just save time, you also gain consistency. When content is broken into smaller units, it becomes easier to adopt that the same terminology, style, and tone throughout the text.
When it comes to automation, segmentation helps systems such as machine translation or automated quality checks process text easier. Nonetheless, the need for segmentation becomes more apparent with complex localization projects and those that demand faster turnaround times.
Types of segmentation
If you’re convinced that segmentation is something you need to add to your localization workflow, you should know the process can vary depending on the language, content type, and purpose. So here are the common types of segmentation you should know about:
Sentence-level segmentation
This is the most widely used approach in translation and localization. In this method, the source text is divided into individual sentences, and each sentence is treated as an independent unit for translation. This approach works well for content that follows a logical and sequential structure (e.g., technical documentation, instructional materials).
Paragraph-level segmentation
This is where the text is divided into entire paragraphs rather than individual sentences like in the first method. Paragraph-level segmentation is useful when the broader context of the paragraph is crucial for understanding the meaning. Best examples would be marketing materials, essays, or creative writing—these often rely on the flow and cohesion of ideas across sentences.
Phrase-level segmentation
This method is typically used when dealing with content that contains complex sentence structures or nuanced expressions. For instance, legal documents, contracts, or poetic works may require this level of segmentation to ensure that every clause or phrase is accurately represented. Translators working with phrase-level segmentation must pay close attention to syntax, semantics, and cultural nuances to maintain the integrity of the original text.
String-level segmentation
Doing segmentation at string-level is common in software localization and involves dividing the text into smaller units, often corresponding to individual strings of code or interface elements. These strings can be anything from user interface components, such as buttons and menus to error messages and notifications. Unlike sentence or paragraph segmentation, string-level segmentation often isolates fragments of text without providing much context. As a result, translators must rely on supplementary materials (screenshots, developer notes) to understand the intended meaning and placement of the strings.
Word-level segmentation
This type of segmentation relies on breaking the text into individual words. It’s less used for general translation projects, but can be valuable for linguistic research, morphological analysis, or creating lexicons. In specialized fields like machine learning, word-level segmentation helps in analyzing language patterns and building models for natural language processing (NLP).
Custom segmentation
What this means is that you are tailoring the segmentation to the unique structure of your content. For example, XML-based documents, scripts, or texts with embedded tags may need to be segmented based on specific markers or metadata.
Final thoughts
Segmentation in localization works by breaking down content into translatable units to improve clarity, efficiency, and consistency throughout the process. You can do it at sentence, paragraph, string, or custom level; the choice of segmentation type depends on the specific needs of the project. Once you master segmentation, you can boost the quality of your translations and create content that resonates with a wider diversity of audiences.