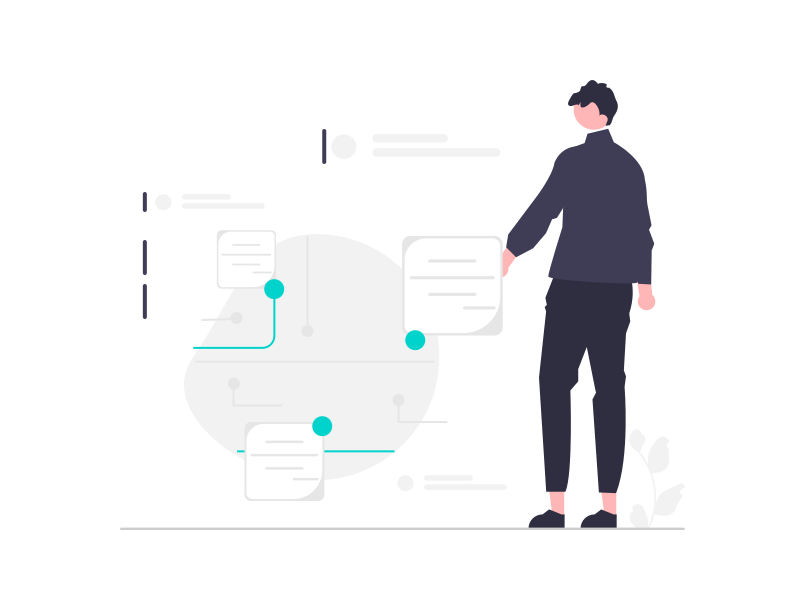
The future of large language models (LLMs) holds exciting possibilities as researchers and developers continue to explore ways to enhance their capabilities, address limitations, and apply them to a variety of fields. Responsible development and deployment practices will play a vital role in shaping the positive impact of large language models in the years to come.
In the context of localization, large language models already play an important role in overcoming linguistic barriers and facilitating effective communication across different languages and cultures. They have the potential to significantly impact and improve the localization process in more ways than one. Below, we discuss the ways in which LLMs can impact and enhance localization efforts.
What is a large language model?
A large language model is a type of artificial intelligence (AI) model that has been trained on a massive amount of text data to understand and generate human-like language. These models are typically based on deep learning architectures, specifically recurrent neural networks (RNNs) or transformer architectures. The term “large” refers to the vast scale of parameters and data used during the training process.
The Cambridge Dictionary defines the LLM as “a complex mathematical representation of language that is based on very large amounts of data and allows computers to produce language that seems similar to what a human might say.”
One of the most well-known examples of a large language model is OpenAI’s GPT (Generative Pre-trained Transformer) series, such as GPT-3. These models have billions of parameters, allowing them to capture complex patterns and relationships within language. The training process involves exposing the model to diverse and extensive datasets, enabling it to learn grammar, context, semantics, and even subtle nuances of language.
Large language models and localization
The integration of large language models in localization signifies a promising future where linguistic and cultural diversity are embraced, communication barriers are dismantled, and businesses can effectively connect with audiences worldwide. LLMs have the potential to significantly impact and improve the localization process in several ways.
Large language models can be employed for high-quality machine translation, helping to translate content accurately and contextually across multiple languages. They can handle nuances, idioms, and cultural references, contributing to more natural and contextually relevant translations. Additionally, these models can assist in adapting content to align with cultural sensitivities and preferences.
Understanding context is crucial for effective localization. Large language models are capable of grasping context from surrounding text, allowing for more context-aware localization. They can also assist in creating and managing glossaries and terminology databases, promoting consistency across translations and adaptations.
For automating certain aspects of the localization workflow, large language models have been found to help streamline the process and reduce the time and effort required for manual tasks. This includes initial content analysis, pre-translation tasks, and even generating suggestions for human translators. In addition, chatbots powered by LLMs can offer multilingual support, addressing user queries and concerns in different languages.
Large language models can not only translate but also generate content in the target language. This can be particularly useful for creating marketing materials, product descriptions, or other content specific to the local audience, tailored to their cultural norms and preferences.
Trends to watch in the coming years
As these powerful AI systems continue to develop, they will play an increasingly important role in helping businesses to localize their content effectively and efficiently. Here’s what we can expect from large language models in the coming years:
- Increased accuracy and coverage. LLMs will continue to improve their accuracy and coverage, making them better able to translate complex and nuanced content. They will also be able to handle a wider range of languages, including less well-resourced languages.
- Enhanced creativity. LLMs will be able to generate more creative and engaging localized content, such as marketing copy, e-learning materials, and customer support scripts. They will also be able to adapt content to different cultures and contexts.
- Integration with existing processes. LLMs will be seamlessly integrated into existing localization workflows, making it easy for businesses to leverage their power. This will involve developing tools and interfaces that make it easy for linguists and other localization professionals to work with LLMs.
- Collaboration between humans and machines. LLMs will not replace human translators, but they will work alongside them to improve the overall quality and efficiency of localization projects. Humans will provide expertise and cultural sensitivity, while LLMs will handle the more routine and labor-intensive tasks.
- Specialization and customization. LLMs will be developed for specific industries and applications, such as healthcare, finance, and e-commerce. This will allow them to provide even more tailored and relevant translations.
Challenges of large language models for localization
Large language models are trained on massive datasets of text data, and these datasets can reflect the biases present in the real world. This means that LLMs may produce biased or inaccurate translations, especially if the target language or culture is not well-represented in the training data. For example, an LLM trained on a dataset of English news articles may produce translations that are biased towards Western perspectives and may not be sensitive to the cultural nuances of the target language.
Despite the fact that large language models are trained on a wide variety of text data, they may not be as effective at translating content that is highly technical or specialized in nature. This is because LLMs may not have been exposed to the specific terminology or nuances of a particular domain. For example, an LLM may be able to translate general-purpose language fluently, but it may struggle with the technical terminology used in a medical or legal document.
Even the best large language models can produce translations that are grammatically incorrect, semantically inaccurate, or culturally insensitive. Therefore, it is important to have human oversight in the localization process. Human translators should review and edit LLM-generated translations to ensure accuracy, fluency, and cultural appropriateness.
Final thoughts
The potential of large language models in localization is vast, ranging from high-quality machine translation that captures context and nuance to context-aware localization that ensures culturally relevant adaptations. The adaptability of these models to regional variations, their efficiency in content generation, and their ability to maintain consistent terminology contribute to a more streamlined and effective localization workflow.
The Translation Technologies Academy has announced their decision to incorporate ChatGPT and LLMs into their curriculum to prepare their students with the relevant knowledge and skills for the dynamic localization industry, as a testament to the growing significance of these technologies. This just goes to show that LLMs are here to stay.
However, as with any powerful technology, the deployment of large language models in localization requires responsible and ethical considerations. Attention must be given to mitigating biases, ensuring cultural sensitivity, and maintaining human oversight to guarantee that the technology serves as a facilitator rather than a potential source of unintended consequences.