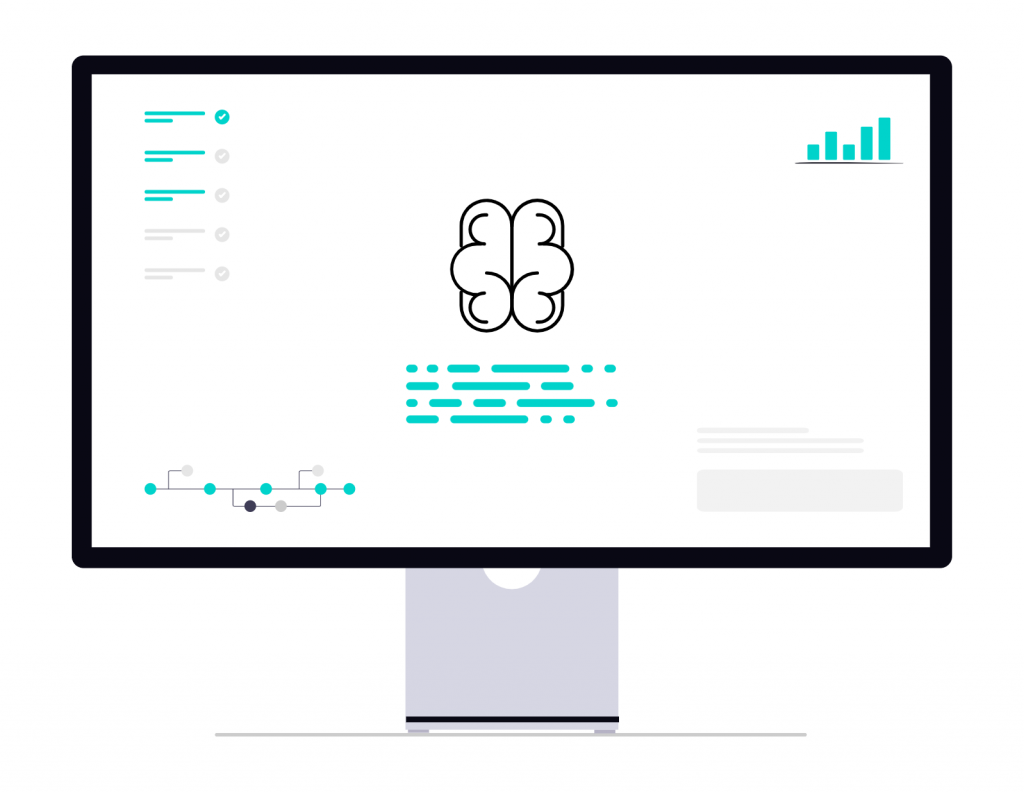
From the earliest rule-based systems to today’s neural networks and transformer architectures, AI has reshaped how machines understand and generate language. The notion of automated translation predates computers, but it was not until the 20th century that it became a serious field of study.
The field has undergone quite a transformation since then. Quite an interesting one, we might say. In this article, we want to trace the historical evolution and impact of AI on machine translation, highlight current technological breakthroughs, and examine the implications and limitations of AI-powered translation systems.
Neural machine translation and the AI revolution
While earlier systems like rule-based and statistical machine translation used logic or probability to mimic aspects of language, they were not truly “intelligent” in the way we understand AI today. Artificial intelligence, particularly in the form of machine learning, began to play a central role in translation starting in the early 2010s. This marked the beginning of neural machine translation (NMT).
NMT systems are built using artificial neural networks, which are inspired by the structure of the human brain. These models are trained on vast amounts of bilingual text and learn to map sequences in one language to sequences in another, capturing semantic relationships and grammatical structures without explicit rules or human-designed features.
The real AI breakthrough came with the introduction of sequence-to-sequence models, which laid the groundwork for using deep learning in language generation tasks. These models, combined with attention mechanisms, significantly improved the ability to translate longer and more complex sentences by focusing on relevant parts of the input during translation.
When Google adopted neural machine translation in 2016 for its Translate platform, it marked a public milestone: machine translation was no longer rule-bound or statistical. Now, it was powered by artificial intelligence in a very real, operational sense. The evolution culminated in 2017 with the introduction of the transformer architecture, which solidified AI’s dominance in the field.
Current capabilities and applications
Modern AI-driven translation systems have achieved near-human performance in many language pairs, particularly among high-resource languages. These systems can translate entire documents while preserving tone, formality, and domain-specific terminology. Fluency and grammaticality have improved to the point where, in everyday usage, AI-generated translations often go unnoticed.
A study published by the Seoul Business School analyzed the translation performances of large language models and NMT systems across various language pairs. The findings indicated that for translations from English to languages like Spanish and French, Google Translate and Microsoft Translator demonstrated superior performance. The study reported BLEU scores of 97% for English-Spanish and 96% for English-French translations, compared to human translation scores of 98% and 96%, respectively.
Real-time speech translation is now a reality, thanks to integrated speech-to-text, translation, and text-to-speech pipelines. Platforms like Zoom, Google Assistant, and Skype offer real-time interpreting functionality. These applications have transformed cross-linguistic communication in business, education, healthcare, and diplomacy.
A particularly important advancement is the move toward multilingual and low-resource translation. Traditional systems required parallel corpora for each language pair, but transformer-based models can learn to translate between languages even when little or no direct training data exists. In 2023, Google added support for 110 new languages, including many African and Indigenous languages.
The issues and challenges
Despite its benefits, AI translation brings some risks, like the phenomenon of hallucination, where models generate fluent but factually incorrect output. Naturally, this is especially dangerous in sensitive domains, where mistranslation can have legal, financial, or health-related consequences.
There are also cultural and ethical issues. We know that AI models are trained on large internet corpora, but these often reproduce the biases found in those datasets. We’re talking problems like gender bias, cultural insensitivity, and underrepresentation of minority dialects.
Some are also concerned about the professional impact AI has on human translators. AI has created new roles but it has also reduced demand for traditional translation work. A study by the Centre for Economic Policy Research (CEPR) found a measurable decline in language-related employment in sectors where machine translation tools were widely adopted.
Conclusion
The impact of AI on machine translation is huge, having transformed it from a rules-based tool into a context-aware system capable of producing fluent and accurate translations across hundreds of languages. The shift to neural networks and transformer architectures has driven unprecedented gains in quality, efficiency, and accessibility.
However, these advances also pose new ethical, professional, and cultural questions that must be addressed as the technology becomes further embedded in global communication. As we look ahead, we can only hope AI translation will not replace human translators, but augment human intelligence.